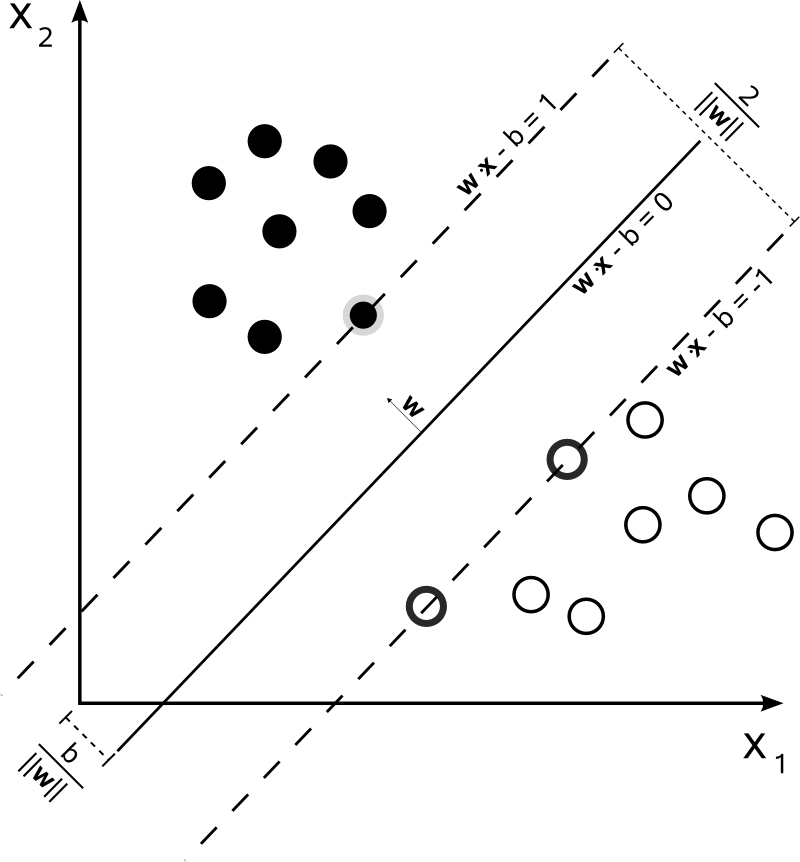
You’ve likely been hearing a lot about something called “Machine Learning” lately, but could this affect 3D printing? Most definitely so!
The concept of machine learning is simply the application of advanced algorithms against previously collected data to develop predications, classifications and decisions about future events. From the outside view it more or less mimics the complex decision making that a human might perform.
We are beginning to see this technology used in real-life applications recently, with much more to come. One notable application is driverless cars, where algorithms and sensors replace the human behind the wheel. While experimental and a novelty at this time, that application will surely knock out many commercial driving jobs from our planet over the next ten years.
And that’s in just one industry; machine learning technology will be applied to countless other areas and combined with robotics may actually cause a severe shortage of jobs for the population.
Meanwhile, how might this technology make its way into 3D printing? I can think of a few ways.
Materials Design: The big focus these days in 3D printing is the development of unique materials that offer advanced engineering properties to match particular situations. It’s possible that machine learning algorithms could assist, simplify and speed up the development of new materials through simulations.
Slicing: 3D printers typically require “sliced” 3D models whose GCODE provides step-by-step instructions for moving the print head to and fro on the build plate. Often such movement patterns are not optimal for the application. They may be inefficient, leading to slower prints, for example. A machine learning algorithm could theoretically optimize the path taken by the print head to simplify the printing process.
Automated Strength: 3D print GCODE paths can also be ineffective: consider a bolt hole that should have extra reinforcement. Some “advanced” slicing programs allow you to manually specify extra material be deposited around that hole. But why not automate the strengthening process? Imagine an intelligent slicer that automatically generates a complex internal fill structure that exactly matches the mechanical stress requirements of the part. Just specify the requirements and the correct part should be produced.
Motion Control: Higher speed 3D printing is sometimes limited by the motion system of a 3D printer that’s pushing and pulling the print head around. Imagine a machine learning system that examined the GCODE path and optimized it to allow for more extreme movements. Instead of simply moving from point A to point B, the system could accelerate to the maximum possible and still slow down at point B for directional changes. In other words, the motion control firmware could essentially be controlled like a Formula 1 driver was at the wheel.
3D Design: Many 3D designs include common components and structures. It’s possible for a machine learning system to anticipate potential structures and provide them for inclusion automatically. This would be analogous to those internet search bars where the search phrase is autocompleted. Why not do that while designing in 3D?
These are a few ideas off the top of my head, and I am very certain there will be many more applications of machine learning in this space.
Maybe you’re thinking of one now? Let us know!
Image Credit: Wikipedia