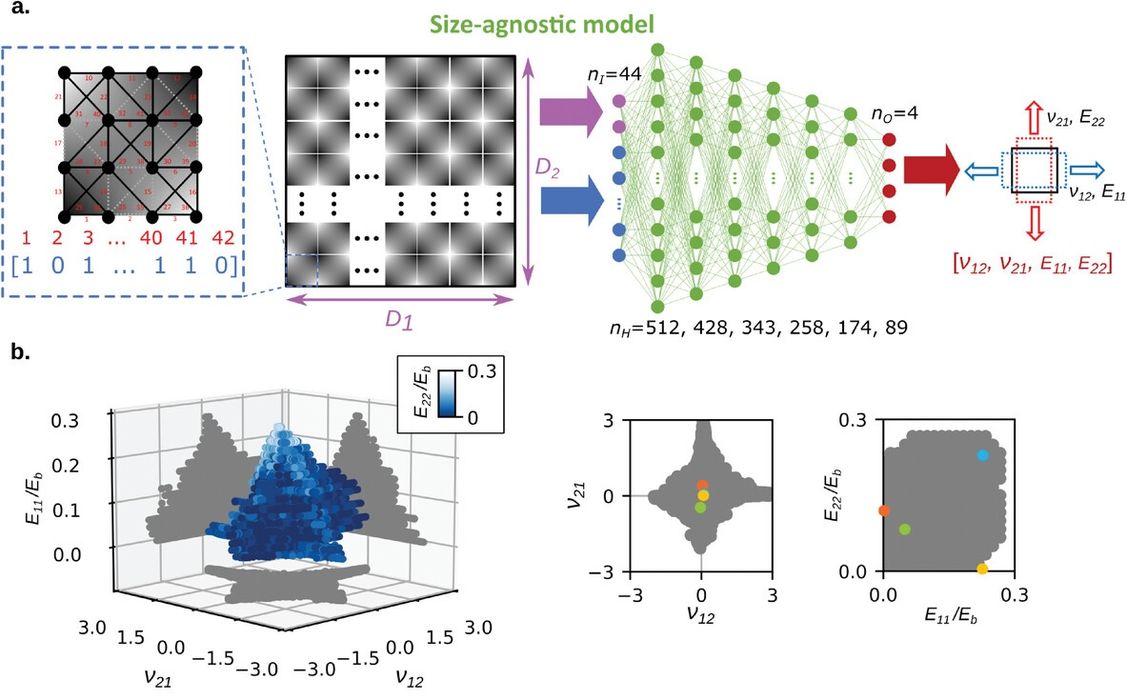
Researchers have developed a powerful method to use AI to generate metamaterial designs that could be 3D printed.
Metamaterials are the secret power of 3D printing. They are (sometimes) complex shapes that exhibit interesting engineering properties simply due to their geometry.
A trivial example of a metamateiral might be a uniform lattice structure. The structure could present flexibility and shock absorbing features, yet itās made of a fairly rigid base material. The shape, rather than the base material, holds the engineering properties.
By combining different types of metamaterials itās possible to design functional objects that are simpler to assemble and require fewer components. In some cases complex subcomponents can be swapped out for metamaterials.
Metamaterials are of considerable interest in 3D printing, because thatās usually the only technology that can reliably produce them at scale.
However, the problem is that metamaterials are challenging to design. One not only has to determine a mechanical structure to implement the desired property, but it has to actually meet physical tests to do so.
This implies a lot of designing, printing, testing and iterations thereof. Thatās expensive, and perhaps is one of the main reasons why metamaterials havenāt taken off.
The new research attempts to overcome this problem through a unique combination of AI techniques.
The researchers were able to develop a deep learning system that was able to very quickly predict the elastic properties of a unit cell structure. Through testing of real 3D printed parts, they verified that the AI simulation was very close to reality.
They then developed another deep learning system called the āunit cell generative modelā to perform inverse design of the unit cells. This output was then fed through the elastic predictor and used to filter out successful generations. They explain:
āWe combined the unit cell generative model and the size-agnostic model to develop a comprehensive and powerful framework called Deep-DRAM, which can inversely design lattice structures with given elastic properties and dimensions.ā
And:
āThe presence of such natural variations in the elastic properties of the generated unit cells enables us to feed a large number of designs created by the deep generative model to the size-agnostic model that is trained to account for the effects of size along each direction. Then, all these generated unit cells together with the desired dimensions are introduced to the size-agnostic model to predict the elastic properties of these combinatorial designs. Finally, the MSE values showing the difference between the target properties and the final elastic properties of the generated combinatorial designs are calculated. Based on the error values, we selected the designs that best matched the target elastic properties for the given dimensions.ā
This is incredible news, as it suggests there may be a way to use AI to generate metamaterial designs on demand. This could be a long-term gamechanger for the 3D printing industry, as thatās the only technology that can properly produce these designs.
One can imagine this technology being incorporated into advanced CAD systems to enable designers to easily generate components made from metamaterial designs. These could be directly integrated into 3D printable objects, greatly simplifying assembly.